LeapFive News
LeapFive Technology Wins the 2024 Science and Technology Award of China Electrotechnical Society
Release time:
2025-01-03
Recently, LeapFive Technology, in collaboration with China Electric Power Research Institute and other partners, was awarded the First Prize of the 2024 Science and Technology Progress Award by the China Electrotechnical Society for its joint research project, “Cross-Modal Intelligent Inspection Technology and Applications for Power IoT Equipment.”
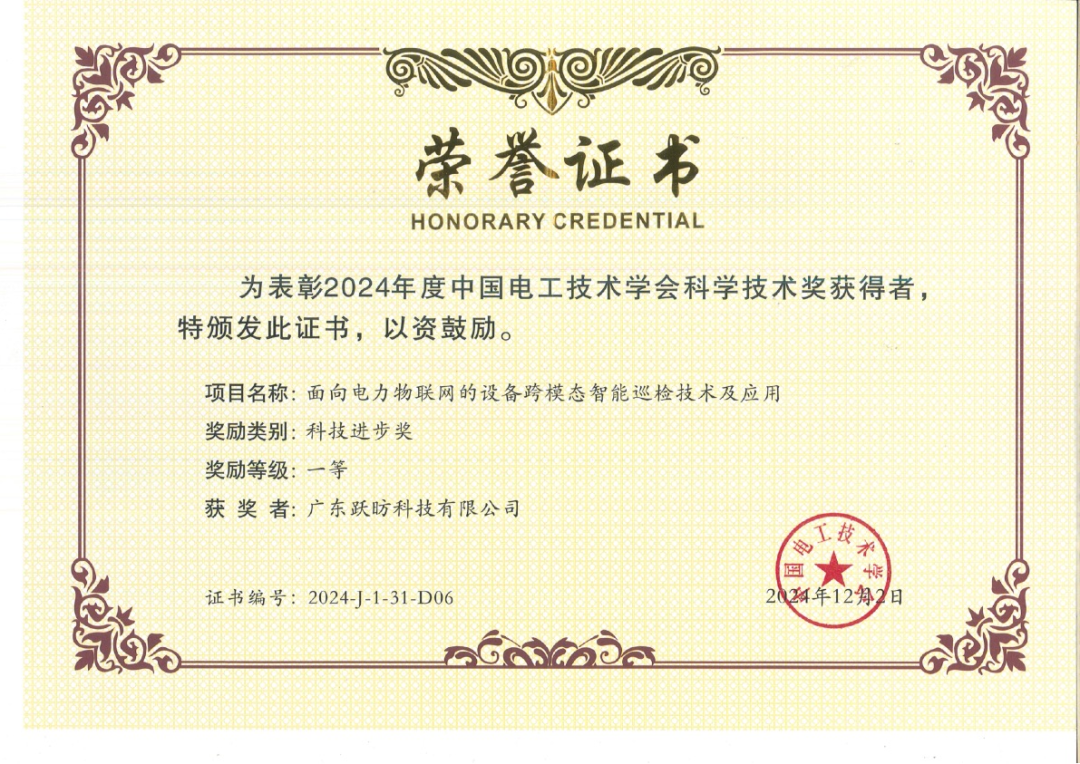
Project Background
With the accelerated construction of next-gen power systems, the scale of equipment has grown exponentially. Utilizing edge-cloud collaborative inference technology enables timely detection of power equipment defects and enhances intelligent inspection capabilities. However, challenges persist in applying this technology to power systems, including:
- High latency and energy consumption of traditional edge computing.
- Inadequate visual recognition accuracy for power equipment defects.
- Difficulty in aligning multi-modal features, affecting fault diagnosis reliability.
- Weak domain knowledge constraints, reducing the accuracy and credibility of ADM (Aid Decision Making) systems.
As a result, intelligent inspection technology for power equipment has faced challenges in achieving widespread adoption.
Key Innovations
Since 2019, LeapFive Technology, in collaboration with China Electric Power Research Institute and other partners, has assembled a project team to address these challenges. The team has developed a series of software and hardware solutions, including RISC-V power edge intelligent gateways, drone-mounted intelligent terminals, and intelligent operation and inspection knowledge management and cognitive reasoning systems. These solutions have been successfully applied in scenarios such as transmission, transformation, and distribution equipment inspections. Key innovations include:
- Optimized Edge Computing for Power Equipment Using Proprietary Chips
- Introduced shift quantization operators to map the storage and computation of edge-side algorithm models to a single hardware platform, significantly reducing data transfer delays and energy consumption.
- Designed RISC-V and computing-in-memory heterogeneous computing logic to decouple general-purpose and specialized computing power, addressing the limited power supply of edge-side intelligent inference terminals.
- Achieved over 3 TOPS/W inference efficiency for edge intelligent terminals through these technological breakthroughs.
- Introduced shift quantization operators to map the storage and computation of edge-side algorithm models to a single hardware platform, significantly reducing data transfer delays and energy consumption.
- Cross-Modal Cognitive Reasoning for Visual Defect Recognition in Power Equipment
- Developed feature-fusion inferencing technology based on self-attention mechanism to guide defect recognition using domain-specific knowledge.
- Enhanced the accuracy of recognizing small targets and irregular features while reducing the need for high-quality training data.
- Improved the recognition rate for 35 complex defect types, such as pin dislocation and transformer corrosion, from 87.2% to 92.6%.
- Developed feature-fusion inferencing technology based on self-attention mechanism to guide defect recognition using domain-specific knowledge.
- Intelligent Fault Diagnosis Using Multi-modal Dynamic Fusion
- Proposed sparse gating strategies for feature alignment and high-order feature extraction across multi-modal sensor data (e.g., acoustic, optical, electrical, and chemical data).
- Designed a self-attention mechanism neural network based on multi-modal dynamic fusion to adaptively assess data confidence from different modalities, enhancing fault diagnosis reliability.
- Increased fault diagnosis accuracy for power equipment from 84% to 93%.
- Proposed sparse gating strategies for feature alignment and high-order feature extraction across multi-modal sensor data (e.g., acoustic, optical, electrical, and chemical data).
- Knowledge-Enhanced ADM for Power Equipment Maintenance
- Integrated domain-specific databases and knowledge graphs to enhance content generation accuracy.
- Developed a context-aware decision generation module constrained by professional power equipment knowledge, overcoming limitations in interpretability of existing ADM systems.
- Improved ADM accuracy for equipment maintenance from 82.3% to 95%.
- Integrated domain-specific databases and knowledge graphs to enhance content generation accuracy.
Achievements and Industry Impact
The project has achieved significant results, including 38 published papers, 2 monographs, 24 authorized patents, 6 software copyrights, and 3 industry standards. An evaluation by the Chinese Society for Electrical Engineering, led by Academician Yunhe Pand, concluded that the project outcomes have reached an internationally leading level. The solutions have been successfully applied in regions such as Tianjin, Zhejiang, Fujian, and Hebei, generating over 440 million CNY in sales revenue over the past three years, with notable economic and social benefits.
As one of the most prestigious and influential awards in China’s electrical engineering field, the Science and Technology Award of China Electrotechnical Society recognizes significant innovations and contributions in the industry. LeapFive Technology’s First Prize achievement underscores its years of technological advancement and innovation, paving the way for broader future growth.
This award is a testament to LeapFive Technology’s innovation strength and a tremendous encouragement for the future. LeapFive Technology will continue to uphold its commitment to open innovation, strengthen its technological capabilities, and collaborate with eco-system partners to drive the intelligent transformation of the power industry.